Built-in color palettes in PIASO#
PIASO provides a diverse selection of discrete color palettes and continous color plattes.
To use the built-in colors in PIASO, you can set the palette=piaso.pl.color.d_color1
for the discrete colors, and cmap=piaso.pl.color.c_color1
for the continuous colors in sc.pl.umap, piaso.pl.plot_embeddings_split or other functions. This notebook aims to display the available choices in both discrete and continuous color maps.
[1]:
path = '/home/vas744/Analysis/Python/Packages/PIASO'
import sys
sys.path.append(path)
[2]:
import piaso
import scanpy as sc
import numpy as np
import matplotlib.pyplot as plt
import warnings
import piaso.plotting.color as color
/home/vas744/.local/lib/python3.9/site-packages/networkx/utils/backends.py:135: RuntimeWarning: networkx backend defined more than once: nx-loopback
backends.update(_get_backends("networkx.backends"))
[3]:
sc.set_figure_params(dpi=80,dpi_save=300, color_map='viridis',facecolor='white')
from matplotlib import rcParams
rcParams['figure.figsize'] = 4, 4
[4]:
warnings.simplefilter(action='ignore', category=FutureWarning)
Load the data#
The 20k subsampled snRNA-seq data from Allen SEA-AD project is available in google drive: https://drive.google.com/file/d/1nH-CRaTQFxJ5pAVpy8_hUQn1nrIcakq2/view?usp=drive_link.
The original data is available in https://portal.brain-map.org/explore/seattle-alzheimers-disease.
[5]:
adata=sc.read('/n/scratch/users/v/vas744/Data/Public/PIASO/SEA-AD_RNA_MTG_subsample_excludeReference_20k_piaso.h5ad')
[6]:
adata
[6]:
AnnData object with n_obs × n_vars = 20000 × 36601
obs: 'sample_id', 'Neurotypical reference', 'Donor ID', 'Organism', 'Brain Region', 'Sex', 'Gender', 'Age at Death', 'Race (choice=White)', 'Race (choice=Black/ African American)', 'Race (choice=Asian)', 'Race (choice=American Indian/ Alaska Native)', 'Race (choice=Native Hawaiian or Pacific Islander)', 'Race (choice=Unknown or unreported)', 'Race (choice=Other)', 'specify other race', 'Hispanic/Latino', 'Highest level of education', 'Years of education', 'PMI', 'Fresh Brain Weight', 'Brain pH', 'Overall AD neuropathological Change', 'Thal', 'Braak', 'CERAD score', 'Overall CAA Score', 'Highest Lewy Body Disease', 'Total Microinfarcts (not observed grossly)', 'Total microinfarcts in screening sections', 'Atherosclerosis', 'Arteriolosclerosis', 'LATE', 'Cognitive Status', 'Last CASI Score', 'Interval from last CASI in months', 'Last MMSE Score', 'Interval from last MMSE in months', 'Last MOCA Score', 'Interval from last MOCA in months', 'APOE Genotype', 'Primary Study Name', 'Secondary Study Name', 'NeuN positive fraction on FANS', 'RIN', 'cell_prep_type', 'facs_population_plan', 'rna_amplification', 'sample_name', 'sample_quantity_count', 'expc_cell_capture', 'method', 'pcr_cycles', 'percent_cdna_longer_than_400bp', 'rna_amplification_pass_fail', 'amplified_quantity_ng', 'load_name', 'library_prep', 'library_input_ng', 'r1_index', 'avg_size_bp', 'quantification_fmol', 'library_prep_pass_fail', 'exp_component_vendor_name', 'batch_vendor_name', 'experiment_component_failed', 'alignment', 'Genome', 'ar_id', 'bc', 'GEX_Estimated_number_of_cells', 'GEX_number_of_reads', 'GEX_sequencing_saturation', 'GEX_Mean_raw_reads_per_cell', 'GEX_Q30_bases_in_barcode', 'GEX_Q30_bases_in_read_2', 'GEX_Q30_bases_in_UMI', 'GEX_Percent_duplicates', 'GEX_Q30_bases_in_sample_index_i1', 'GEX_Q30_bases_in_sample_index_i2', 'GEX_Reads_with_TSO', 'GEX_Sequenced_read_pairs', 'GEX_Valid_UMIs', 'GEX_Valid_barcodes', 'GEX_Reads_mapped_to_genome', 'GEX_Reads_mapped_confidently_to_genome', 'GEX_Reads_mapped_confidently_to_intergenic_regions', 'GEX_Reads_mapped_confidently_to_intronic_regions', 'GEX_Reads_mapped_confidently_to_exonic_regions', 'GEX_Reads_mapped_confidently_to_transcriptome', 'GEX_Reads_mapped_antisense_to_gene', 'GEX_Fraction_of_transcriptomic_reads_in_cells', 'GEX_Total_genes_detected', 'GEX_Median_UMI_counts_per_cell', 'GEX_Median_genes_per_cell', 'Multiome_Feature_linkages_detected', 'Multiome_Linked_genes', 'Multiome_Linked_peaks', 'ATAC_Confidently_mapped_read_pairs', 'ATAC_Fraction_of_genome_in_peaks', 'ATAC_Fraction_of_high_quality_fragments_in_cells', 'ATAC_Fraction_of_high_quality_fragments_overlapping_TSS', 'ATAC_Fraction_of_high_quality_fragments_overlapping_peaks', 'ATAC_Fraction_of_transposition_events_in_peaks_in_cells', 'ATAC_Mean_raw_read_pairs_per_cell', 'ATAC_Median_high_quality_fragments_per_cell', 'ATAC_Non-nuclear_read_pairs', 'ATAC_Number_of_peaks', 'ATAC_Percent_duplicates', 'ATAC_Q30_bases_in_barcode', 'ATAC_Q30_bases_in_read_1', 'ATAC_Q30_bases_in_read_2', 'ATAC_Q30_bases_in_sample_index_i1', 'ATAC_Sequenced_read_pairs', 'ATAC_TSS_enrichment_score', 'ATAC_Unmapped_read_pairs', 'ATAC_Valid_barcodes', 'Number of mapped reads', 'Number of unmapped reads', 'Number of multimapped reads', 'Number of reads', 'Number of UMIs', 'Genes detected', 'Doublet score', 'Fraction mitochondrial UMIs', 'Used in analysis', 'Class confidence', 'Class', 'Subclass confidence', 'Subclass', 'Supertype confidence', 'Supertype (non-expanded)', 'Supertype', 'Continuous Pseudo-progression Score', 'Severely Affected Donor'
var: 'gene_ids'
uns: 'APOE4 Status_colors', 'Braak_colors', 'CERAD score_colors', 'Cognitive Status_colors', 'Great Apes Metadata', 'Highest Lewy Body Disease_colors', 'LATE_colors', 'Overall AD neuropathological Change_colors', 'Sex_colors', 'Subclass_colors', 'Supertype_colors', 'Thal_colors', 'UW Clinical Metadata', 'X_normalization', 'batch_condition', 'default_embedding', 'neighbors', 'title', 'umap'
obsm: 'X_scVI', 'X_umap'
layers: 'UMIs'
obsp: 'connectivities', 'distances'
Discrete color palettes#
[7]:
palettes = [c for c in vars(color) if c.startswith('d_')]
n = len(palettes)
ncol = 4
nrow = None
row_size, col_size = 2.4, 2.4
if ncol is None:
nrow = int(np.sqrt(n))
ncol = int(np.ceil(n / nrow))
else:
nrow = int(np.ceil(n / ncol))
fig, axs = plt.subplots(nrow, ncol, dpi=96, figsize=(ncol * col_size, nrow * row_size))
c = 0
for i in range(len(axs)):
for j in range(len(axs[0])):
if c < n:
palette_value = getattr(color, palettes[c], None)
sc.pl.embedding(adata,
basis='X_umap',
color=['Subclass'],
palette=palette_value,
cmap='Spectral_r',
size=3,
frameon=False,
ax=axs[i][j],
title='piaso.plotting.color.'+str(palettes[c]+f' - {len(getattr(color, palettes[c], None))} colors'),
legend_loc=None,
show=False)
axs[i][j].title.set_size(9)
else:
axs[i][j].set_visible(False)
c += 1
plt.suptitle("Selection of discrete color palettes")
plt.tight_layout()
plt.show()
WARNING: Length of palette colors is smaller than the number of categories (palette length: 19, categories length: 24. Some categories will have the same color.
WARNING: Length of palette colors is smaller than the number of categories (palette length: 13, categories length: 24. Some categories will have the same color.
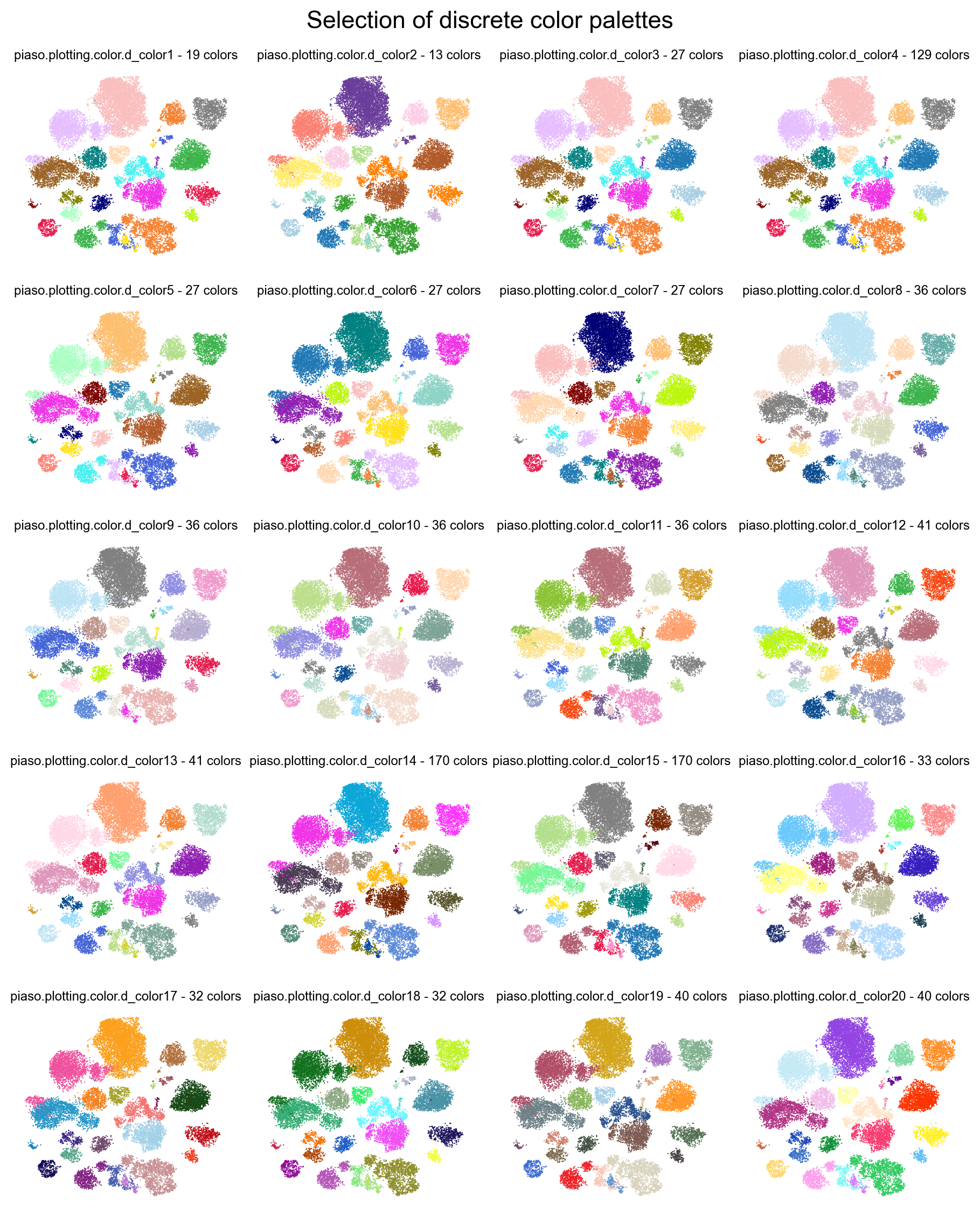
Continuous color palettes#
[8]:
palettes = [c for c in vars(color) if c.startswith('c_')]
n = len(palettes)
ncol = None
nrow = None
row_size, col_size = 2.3, 2.3
if ncol is None:
nrow = int(np.sqrt(n))
ncol = int(np.ceil(n / nrow))
else:
nrow = int(np.ceil(n / ncol))
fig, axs = plt.subplots(nrow, ncol, dpi=96, figsize=(ncol * col_size, nrow * row_size))
c = 0
for i in range(len(axs)):
for j in range(len(axs[0])):
if c < n:
palette_value = getattr(color, palettes[c], None)
sc.pl.umap(adata,
color=['GAD1'],
cmap=palette_value,
size=15,
frameon=False,
ax=axs[i][j],
title='piaso.plotting.color.'+str(palettes[c]),
legend_loc=None,
show=False)
axs[i][j].title.set_size(9)
else:
axs[i][j].set_visible(False)
c += 1
plt.suptitle("Selection of continuous color palettes")
plt.tight_layout()
plt.show()
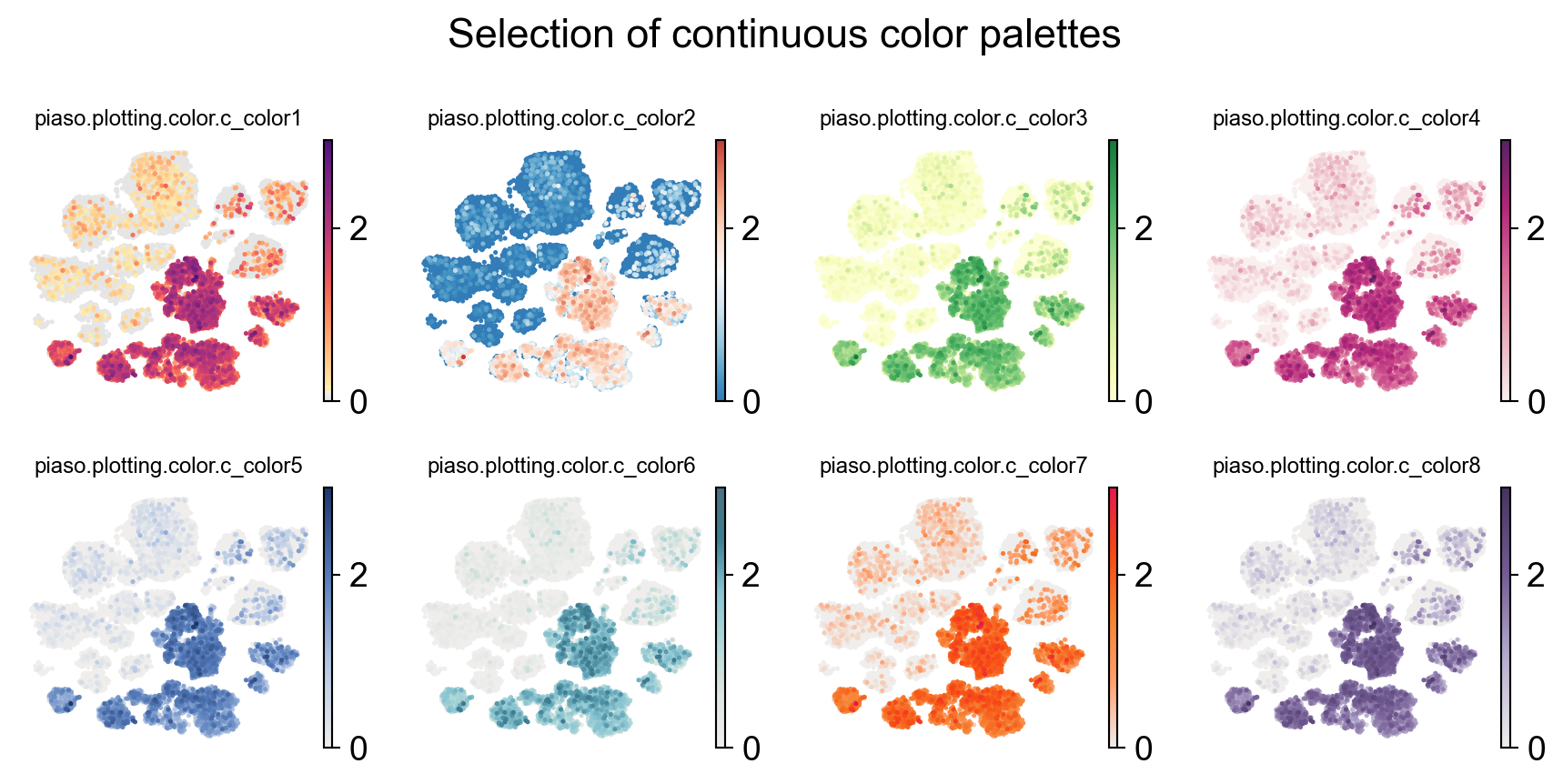